Risk adjustment in audit of outcome after head and neck surgery applied to cumulative sum chart methodology to monitor of free flap failure
“Soon, there will be a time where our scholars & colleagues will not be satisfied with general comments on surgical quality outcomes—instead, they will call any physician charlatan who is incapable to quantify his results.”—Theodore Billroth 1860
Introduction
Surgeons’ efforts to audit post-operative patient outcomes, in order to measure quality of care systematically, have increased over recent years. National Audits, within the National Clinical Audit Patient Outcomes Program (NCAPOP) provide information on quality of surgical care. The annual reports produced by the National Audits produce are accessible to the public. Cardiothoracic surgeons led the modern era of national audit in a major response to the Bristol Royal Infirmary Inquiry into Paediatric Heart Surgery in the 1980’s and 1990’s (1). The Inquiry investigated increased mortality rate in the Cardiothoracic unit at this hospital but had explicit implications for the entire NHS. The government response that followed, “Learning from Bristol” was a landmark paper and called for new standards of care, openness and monitoring (2). It highlighted a lack of published standards of care, lack of information made available to patients and relatives using the services, and lack of external ongoing scrutiny of performance. Over this time-period, in many areas of society, computational intensive techniques known as ‘machine-learning’ were developed and applied to complex problems to guide governance and aid decision-making. The same is occurring in medical science, and in particular, surgeon-led audit.
Metric choice
It is argued that in order for the outcome or metric to be effective they must be usable (the information can be actioned and understood), feasible (the data can be collected and measured), reproducible, meaningful (the metrics are agreed on by stakeholders), promote quality improvement (metrics can be monitored), and possess face validity (expert consensus exists that there will be an association with improved outcomes). We argue metrics must also be selected that can be modelled in order that risk-adjustment, which takes account of variation in complexity of individual patients, is possible.
By way of example, the Society of Cardio-Thoracic Surgery has published 10 risk adjustment algorithms since the first models (Euro Score, Euro Score II) which were embedded in national (and international) audit (3). This trend is continuing in other surgical specialities as National Audits mature. The online library of medical algorithms, MedicalAl has 186 post-operative complication prediction algorithms that can be used for audit (4).
Decisions about pertinent metrics in the fields of Oral and Maxillofacial Surgery are being made in the UK and elsewhere where national quality improvement programmes exist, such as the National Clinical Improvement Programme in the UK (NCIP), Quality and Outcomes in Oral & Maxillofacial Surgery programme in the UK (QOMS) and the American College of Surgeons National Surgical Quality Improvement Programme (ACS NSQIP) in the US. As of 2020, these three programmes have chosen the following metrics in the field of Head & Neck Oncology and Reconstruction (Table 1).
Table 1
NCIP | QOMS | ACS NSQIP | |
---|---|---|---|
H & N oncology | Return to theatre within 30 days | Serious complication | Serious complication |
Readmission with 30 days | Lymph nodes in a neck dissection (>18) | Any complication | |
Workload/year | Positivity of surgical margins | Pneumonia | |
Reconstruction | Length of hospital stay | Cardiac complication | |
Free-flap failure | Surgical site infection | ||
Delay to radiotherapy >42 days | Urinary tract infection | ||
Venous thromboembolism | |||
Renal failure | |||
Sepsis | |||
Readmission | |||
Return to theatre | |||
Death | |||
Discharge to rehab or nursing facility |
NCIP, National Clinical Improvement Programme; QOMS, Quality Outcomes in Oral and Maxillofacial Surgery; ACS NSQIP, American College of Surgeons National Surgical Quality Improvement Programme.
These metrics taken together represent a ‘care quality signature’ which should demonstrate to patients and peers the ongoing performance of a surgical unit. An early example of a proponent of ‘clinical care signature’ is from the US, which reported on 19 separate metrics and later correlated them with overall survival. The following metrics were associated with increased survival: lymph node count of 18 nodes or more in an elective neck dissection, no 30-day non-elective readmissions, and referral for post-operative radiotherapy for stage III or IV disease (5).
Statistical & machine learning techniques
Multivariable regression analyses are standard techniques to analyse outcome data in medical datasets by identifying independent relationships between patient characteristics and a dependent variable.
A simple linear regression model has a single continuous outcome and a single predictor, whereas a multiple or multivariable linear regression model has a single continuous outcome and multiple predictors (continuous or categorical). A simple linear regression model takes the form:
A multivariable or multiple linear regression model takes the form:
where y is a continuous dependent variable, x is a single predictor in the simple regression model, and are the predictors in the multi variable model. In a multi-variable logistic regression model the dependent variable is dichotomous, or binary and the range of predicted probabilities form a sigmoidal curve. A multi-variable linear regression model would be suitable for length of hospital stay (number of days) whereas a multi variable logistic regression model would be suitable for ‘complication YES/NO’ or free flap failure YES/NO models.
The weaknesses of these techniques are numerous and can be found in different sources (6). Dealing with ‘missingness’ in the data is complex, as the equation will not produce an output prediction without all fields being present, and in clinical datasets this can lead to a loss of power at an early stage of analysis. Also, linear relationships will be readily identified, whereas non-linear relationships, which often exist in physiology and medicine, will not be identified by this technique.
An alternative statistical method that has regained interest in medical data-set analysis is Bayesian analysis based on the following probability equation, and developed into a method by the Reverend Thomas Bayes in 1790 (7).
Where ‘x’ is the variable of interest, conditional on ‘y’ the known variable, and P (x) represents prior probability, p (y) is new evidence, and P (y|x) is the likelihood ratio. In terms of making statements about probability of an event, if that event is non-repeatable then strictly probability based on known frequency is impossible to generate. This fundamental concept does not limit Bayesian analysis because (pY) or ‘prior knowledge’ can be subjective including ‘expert’ opinion, which provides a general intuition about the probability of a ‘one-off’ event and can be mathematically combined with other data (as shown) to generate a ‘the posterior probability,’ P (x|y). Strictly, the variables in a multivariate Bayesian analysis need to be independent with no interaction.
Decision tree analysis, artificial neural networks, random forests have also been applied to datasets which are similar to those being studied in this paper. There advantages and disadvantages are beyond the scope this paper but they seek the same aim; to correctly classify (predict) a chosen outcome dependent on patient risk-factors.
Classification performance can be reported in terms of discrimination, calibration and accuracy. Principle among these are: the ‘goodness of fit statistic’ (Hosmer-Lemeshow); the area under the curve; the accuracy, precision and recall and the Brier’s score. The definitions are in the Appendix 1. We use these methods to report predictive performance of our risk-adjustment algorithms.
Methods
A combined dataset of 1,316 patients from 6 NHS units was developed (Author 1). At the stage of writing this dataset has been combined with a further 2 NHS units, 63 care episodes from the second cohort and 1,016 from a third cohort (Author 3). All patients received surgery with curative intent for head and neck squamous cell carcinoma (HNSCC) and had immediate free tissue transfer under general anaesthesia. The datasets include cases done by otolaryngology colleagues where free-tissue transfer was required. All audit datasets were registered with the respective hospital trust clinical audit departments. Ethical approval from was given from the author’s NHS Trust under the ‘Grey Area Project’ process as the published results of this multi-centre audit could be considered generalizable. Patient demographics, co-morbidity using the ACE-27 index, indices of functional status namely the WHO (World Health Organisation) performance status; tumour stage (TNM status, AJCC v7) and operative and anaesthetic treatment were recorded. The ‘high-risk’ variable is a binary field derived from the OPCv4 (Operation Procedure Codes, Version 4) to include any procedure which required oral, pharyngeal or laryngeal mucosal suturing in association with a neck dissection that could lead to saliva escape. Data was pre-processed by the lead author in Microsoft Excel [2013] and analysed in MedCalc v19.1 and Waikato Learning Environment for Knowledge analysis (WEKA) v 3.8.3. Complications were classified using the Clavien-Dindo classification system (8), length of stay was defined as date of operation to date of discharge from hospital, and positivity of margins was classified as <1 mm, using the Royal College of Pathologists definition (9).
Initial exploratory experiments were done including univariate analyses of categorical variables with Chi squared tests and Analysis of Variance (ANOVA) for continuous variables choosing a significance level of P≤0.05). We tested many multi-variable methodologies in MedCalc and WEKA. The data was split into a training-set (70%) and test-set (30%) for development of the earliest published models, namely length of hospital stay model and 30 day complication models. We used 10-fold cross validation as a more robust, less optimistic method in the later publications reporting machine learning algorithms, which were developed on the WEKA platform. The C-statistic was used as a means of comparing model discrimination and to choose the best model. We summarise results by presenting the ‘champion models’ of four metrics: complications within 30 days; severe complications (Clavien-Dindo >3) within 30 days; length of hospital stay (days); and positivity of surgical margins (Table 2). Further details, including calibration test results, are included in their respective publications (10-12) and model outputs (Tables S1-S3, Figure S1).
Table 2
Outcome | Classifier | Sensitivity | Specificity | Accuracy | C statistic | Confusion matrix | |
---|---|---|---|---|---|---|---|
Predicted 0 | Predicted 1 | ||||||
Complication with 30 days | Neural Net | 0.82 | 0.75 | 0.78 | 0.85 | 105 | 39 |
23 | 118 | ||||||
Severe complication within 30 days | Random Forest | 0.85 | 0.73 | 0.85 | 0.79 | 1,110 | 3 |
191 | 8 | ||||||
Length of hospital stay <15 days | Decision Tree | 0.8 | 0.78 | 0.8 | 0.77 | 484 | 33 |
104 | 90 | ||||||
Positivity of surgical margins | Bayes Classifier | 0.58 | 0.77 | 0.75 | 0.7 | 66 | 230 |
50 | 768 |
HNSCC, head and neck squamous cell carcinoma.
For a new phase in the analysis we attempted to include data from a two units (n=63) and (n=1,109). Using the combined dataset we attempted to develop a new pilot risk adjustment model on ‘complete flap failure’ as the primary outcome. Free flap failure was defined as post-anastomotic irreversible loss of flap viability due to ischaemia. We (again) investigated univariate relationships in MedCalc then tested machine-learning algorithms in WEKA, comparing their discrimination and calibration.
We present flap failure loss against time in cumulative sum charts (CuSUM), a form of statistical process control. We embed the risk-adjustment algorithm into the CuSUM methodology as done by Rasmussen et al. (13) but using free flap failure instead of 30-day mortality as the outcome measure.
Results
Of a total 1,593 care episodes there were 76 (4.7%) complete free flap failures in individual patients, and 34 (2%) incidence of partial flap failure. There were significant differences in the prevalence of risk-factors between treating units underlining the importance of risk stratification (Table 3). On univariate analysis there was no significant difference between free flap failure rates and treating hospitals (Group 1, 6%; Group 2, 6%; Group 3, 5%; Group 4, 8%; Group 5, 3%; Group 6, 3%; Group 7, 5%; Group 8, 5%; λ2 3.4, P=0.8) or demographic; alcohol or smoking history; past medical history of arteriosclerosis related diseases including diabetes, ACE-27, WHO performance status; or use of tracheostomy. There were significant association found between primary tumour site (λ2 33.9, P=0.001), use of double flaps (λ2 9.9, P=0.001), use of radial free forearm flaps (λ2 6.3, P=0.01), use of latissimus dorsi (λ2 7.8, P=0.005) and subscapular system flaps (λ2 4.8, P=0.03), previous radiotherapy to the operative site (λ2 5.7, P=0.05), previous surgery (λ2 6.3, P=0.04) and T classification of the tumour (λ2 13.4, P=0.02) and free flap failure. The N classification (λ2 11.1, P=0.08) had a non-significant association. Finally, an unexpected finding was that ‘high-risk’ status had a significantly lower chance of being associated with free flap failure (λ2 7.4, P=0.006) and further scrutiny suggests that midface skin, sinus and skull base pathology are significantly associated with flap failure on univariate analysis (https://cdn.amegroups.cn/static/public/FOMM-2020-HNR-04-1.xls). Notable absence of several independent factors needed for further modelling meant data from Hospital 7 was excluded from further stages of the analysis.
Table 3
Variables | Hospital (group) | Total | F ratio | P value | |||||||
---|---|---|---|---|---|---|---|---|---|---|---|
1 | 2 | 3 | 4 | 5 | 6 | 7 | 8 | ||||
Age | 33 | 38 | 141 | 97 | 33 | 102 | 63 | 1,108 | 4.123 | <000.1 | |
Mean | 66.7879 | 65.7368 | 59.5035 | 63.1443 | 60.1703 | 63.9804 | 56.2381 | 61.3628 | |||
1 SD | 12.9585 | 11.7351 | 13.1076 | 12.9261 | 13.6023 | 11.8497 | 15.4196 | 12.9701 | |||
Gender | |||||||||||
Male | 22 | 24 | 86 | 70 | 20 | 79 | 45 | 520 | 866 (53.3%) | <0.001 | |
Female | 11 | 15 | 58 | 25 | 13 | 29 | 18 | 589 | 758 (46.7%) | ||
Total | 33 (2.0%) | 39 (2.4%) | 144 (8.9%) | 95 (5.8%) | 33 (2.0%) | 108 (6.7%) | 63 (3.9%) | 1,109 (68.3%) | 1,624 | ||
Alcohol | |||||||||||
Never | 10 | 19 | 10 | 22 | 11 | 10 | 0 | 489 | 571 (35.7%) | 914.928 | <0.001 |
Mild | 2 | 8 | 59 | 27 | 9 | 39 | 0 | 212 | 356 (22.3%) | ||
Moderate | 0 | 2 | 13 | 20 | 9 | 32 | 0 | 177 | 253 (15.8%) | ||
Heavy | 13 | 5 | 36 | 18 | 2 | 14 | 0 | 130 | 218 (13.6%) | ||
Ex-heavy | 0 | 3 | 11 | 8 | 2 | 13 | 0 | 4 | 41 (2.6%) | ||
Missing | 0 | 0 | 0 | 0 | 0 | 0 | 63 | 97 | 160 (10.0%) | ||
Total | 25 (1.6%) | 37 (2.3%) | 129 (8.1%) | 95 (5.9%) | 33 (2.1%) | 108 (6.8%) | 63 (3.9%) | 1,109 (69.4%) | 1,599 | ||
Smoking | |||||||||||
Never | 7 | 17 | 34 | 37 | 6 | 82 | 0 | 646 | 829 (52.0%) | 1655.708 | <0.001 |
Ex or current | 17 | 22 | 99 | 58 | 27 | 26 | 0 | 451 | 700 (43.9%) | ||
Missing | 0 | 0 | 0 | 0 | 0 | 0 | 63 | 2 | 65 (4.1%) | ||
Total | 24 (1.5%) | 39 (2.4%) | 133 (8.3%) | 95 (6.0%) | 33 (2.1%) | 108 (6.8%) | 63 (4.0%) | 1,099 (68.9%) | 1,594 | ||
ACE-27 | |||||||||||
0 | 16 | 0 | 71 | 25 | 15 | 33 | 0 | 112 | 272 (16.7%) | 606.481 | <0.001 |
1 | 9 | 30 | 47 | 52 | 13 | 54 | 0 | 205 | 410 (25.2%) | ||
2 | 5 | 9 | 13 | 15 | 5 | 11 | 0 | 124 | 182 (11.2%) | ||
3 | 1 | 0 | 0 | 3 | 0 | 4 | 0 | 26 | 34 (2.1%) | ||
Missing | 2 | 0 | 13 | 0 | 0 | 6 | 63 | 642 | 726 (44.7%) | ||
Total | 33 (2.0%) | 39 (2.4%) | 144 (8.9%) | 95 (5.8%) | 33 (2.0%) | 108 (6.7%) | 63 (3.9%) | 1,109 (68.3%) | 1,624 | ||
ASA | |||||||||||
0 | 0 | 0 | 0 | 1 | 0 | 5 | 0 | 1 | 7 (0.4%) | 1021.474 | <0.001 |
1 | 10 | 6 | 0 | 15 | 3 | 6 | 0 | 94 | 134 (8.3%) | ||
2 | 9 | 26 | 0 | 57 | 25 | 64 | 0 | 463 | 644 (39.7%) | ||
3 | 10 | 7 | 0 | 22 | 5 | 25 | 0 | 417 | 486 (29.9%) | ||
4 | 1 | 0 | 0 | 0 | 0 | 1 | 0 | 7 | 9 (0.6%) | ||
Missing | 3 | 0 | 144 | 0 | 0 | 7 | 63 | 127 | 344 (21.2%) | ||
Total | 33 (2.0%) | 39 (2.4%) | 144 (8.9%) | 95 (5.8%) | 33 (2.0%) | 108 (6.7%) | 63 (3.9%) | 1,109 (68.3%) | 1,624 | ||
WHO Status | |||||||||||
0 | 5 | 4 | 107 | 67 | 11 | 32 | 0 | 611 | 837 (51.5%) | 1007.449 | <0.001 |
1 | 20 | 30 | 22 | 19 | 21 | 48 | 0 | 371 | 531 (32.7%) | ||
2 | 4 | 5 | 5 | 8 | 1 | 18 | 0 | 82 | 123 (7.6%) | ||
3 | 2 | 0 | 1 | 1 | 0 | 3 | 0 | 10 | 17 (1.0%) | ||
Missing | 2 | 0 | 9 | 0 | 0 | 7 | 63 | 35 | 116 (7.1%) | ||
Total | 33 (2.0%) | 39 (2.4%) | 144 (8.9%) | 95 (5.8%) | 33 (2.0%) | 108 (6.7%) | 63 (3.9%) | 1,109 (68.3%) | 1,624 | ||
Diabetes | |||||||||||
0 | 33 | 36 | 143 | 93 | 32 | 100 | 0 | 1,022 | 1,459 (89.8%) | 162.105 | <0.001 |
1 | 0 | 3 | 1 | 2 | 1 | 8 | 0 | 87 | 102 (6.3%) | ||
Missing | 0 | 0 | 0 | 0 | 0 | 0 | 63 | 0 | 63 (3.9%) | ||
Total | 33 (2.0%) | 39 (2.4%) | 144 (8.9%) | 95 (5.8%) | 33 (2.0%) | 108 (6.7%) | 63 (3.9%) | 1,109 (68.3%) | 1,624 | ||
Peripheral vascular disease | |||||||||||
0 | 32 | 38 | 141 | 94 | 32 | 104 | 63 | 1,038 | 1,542 (95.0%) | 14.863 | P = 0.0378 |
1 | 1 | 1 | 3 | 1 | 1 | 4 | 0 | 71 | 82 (5.0%) | ||
Total | 33 (2.0%) | 39 (2.4%) | 144 (8.9%) | 95 (5.8%) | 33 (2.0%) | 108 (6.7%) | 63 (3.9%) | 1,109 (68.3%) | 1,624 | ||
Previous radiotherapy | |||||||||||
Yes | 3 | 4 | 0 | 11 | 12 | 25 | 0 | 183 | 238 (14.7%) | ||
Missing | 2 | 0 | 10 | 0 | 21 | 0 | 63 | 0 | 96 (5.9%) | ||
Total | 33 (2.0%) | 39 (2.4%) | 144 (8.9%) | 95 (5.8%) | 33 (2.0%) | 108 (6.7%) | 63 (3.9%) | 1,109 (68.3%) | 1,624 | ||
Previous surgery | |||||||||||
No | 29 | 30 | 121 | 77 | 0 | 84 | 0 | 845 | 1,186 (73.0%) | 1320.557 | <0.001 |
Yes | 2 | 9 | 13 | 18 | 12 | 24 | 0 | 264 | 342 (21.1%) | ||
Missing | 2 | 0 | 10 | 0 | 21 | 0 | 63 | 0 | 96 (5.9%) | ||
Total | 33 (2.0%) | 39 (2.4%) | 144 (8.9%) | 95 (5.8%) | 33 (2.0%) | 108 (6.7%) | 63 (3.9%) | 1,109 (68.3%) | 1,624 | ||
High risk | |||||||||||
No | 7 | 16 | 37 | 43 | 1 | 17 | 26 | 260 | 407 (25.1%) | 50.108 | <0.001 |
Yes | 26 | 23 | 107 | 52 | 32 | 91 | 37 | 849 | 1,217 (74.9%) | ||
Total | 33 (2.0%) | 39 (2.4%) | 144 (8.9%) | 95 (5.8%) | 33 (2.0%) | 108 (6.7%) | 63 (3.9%) | 1,109 (68.3%) | 1,624 | ||
T Classification | |||||||||||
0 | 0 | 0 | 3 | 4 | 1 | 7 | 0 | 2 | 17 (1.0%) | 503.997 | <0.001 |
1 | 4 | 7 | 8 | 15 | 6 | 7 | 0 | 87 | 134 (8.3%) | ||
2 | 10 | 15 | 56 | 21 | 13 | 20 | 0 | 167 | 302 (18.6%) | ||
3 | 3 | 5 | 35 | 6 | 1 | 12 | 0 | 73 | 135 (8.3%) | ||
4 | 15 | 11 | 35 | 48 | 12 | 60 | 0 | 301 | 482 (29.7%) | ||
Missing | 1 | 1 | 7 | 1 | 0 | 2 | 63 | 479 | 554 (34.1%) | ||
Total | 33 (2.0%) | 39 (2.4%) | 144 (8.9%) | 95 (5.8%) | 33 (2.0%) | 108 (6.7%) | 63 (3.9%) | 1,109 (68.3%) | 1,624 | ||
N Classification | |||||||||||
0 | 19 | 19 | 88 | 52 | 19 | 53 | 0 | 356 | 606 (37.3%) | 823.443 | <0.001 |
1 | 5 | 8 | 38 | 11 | 5 | 12 | 0 | 78 | 157 (9.7%) | ||
2 | 1 | 3 | 6 | 1 | 0 | 2 | 0 | 164 | 177 (10.9%) | ||
3 | 7 | 5 | 3 | 25 | 7 | 20 | 0 | 4 | 71 (4.4%) | ||
4 | 0 | 1 | 1 | 2 | 0 | 10 | 0 | 2 | 16 (1.0%) | ||
? | 1 | 3 | 6 | 2 | 2 | 0 | 63 | 497 | 574 (35.3%) | ||
Total | 33 (2.0%) | 39 (2.4%) | 144 (8.9%) | 95 (5.8%) | 33 (2.0%) | 108 (6.7%) | 63 (3.9%) | 1,109 (68.3%) | 1,624 | ||
Site of tumour | |||||||||||
1 | 0 | 0 | 8 | 0 | 0 | 0 | 0 | 7 | 15 (0.9%) | 269.277 | <0.001 |
2 | 27 | 37 | 122 | 82 | 27 | 79 | 47 | 720 | 1,141 (70.3%) | ||
3 | 1 | 1 | 10 | 1 | 4 | 2 | 0 | 102 | 121 (7.5%) | ||
4 | 0 | 0 | 0 | 1 | 0 | 0 | 0 | 2 | 3 (0.2%) | ||
5 | 1 | 0 | 0 | 0 | 0 | 2 | 0 | 4 | 7 (0.4%) | ||
6 | 0 | 0 | 0 | 0 | 0 | 1 | 0 | 26 | 27 (1.7%) | ||
7 | 4 | 0 | 0 | 3 | 2 | 10 | 1 | 18 | 38 (2.3%) | ||
9 | 0 | 0 | 0 | 3 | 0 | 3 | 4 | 96 | 106 (6.5%) | ||
10 | 0 | 0 | 4 | 2 | 0 | 0 | 1 | 1 | 8 (0.5%) | ||
11 | 0 | 0 | 0 | 2 | 0 | 5 | 3 | 44 | 54 (3.3%) | ||
12 | 0 | 1 | 0 | 1 | 0 | 0 | 7 | 65 | 74 (4.6%) | ||
Missing | 0 | 0 | 0 | 0 | 0 | 6 | 0 | 24 | 30 (1.9%) | ||
Total | 33 (2.0%) | 39 (2.4%) | 144 (8.9%) | 95 (5.8%) | 33 (2.0%) | 109 (6.7%) | 63 (3.9%) | 1,110 (68.3%) | 1,624 | ||
Bilateral neck | |||||||||||
No | 33 | 39 | 144 | 95 | 33 | 108 | 56 | 962 | 1470 (90.5%) | 65.934 | <0.001 |
Yes | 0 | 0 | 0 | 0 | 0 | 0 | 7 | 147 | 154 (9.5%) | ||
Total | 33 (2.0%) | 39 (2.4%) | 144 (8.9%) | 95 (5.8%) | 33 (2.0%) | 108 (6.7%) | 63 (3.9%) | 1,109 (68.3%) | 1,624 | ||
Composite flap | |||||||||||
No | 30 | 30 | 116 | 88 | 29 | 108 | 47 | 777 | 1,225 (75.4%) | 76.721 | <0.001 |
Yes | 3 | 9 | 28 | 7 | 4 | 0 | 16 | 332 | 399 (24.6%) | ||
Total | 33 (2.0%) | 39 (2.4%) | 144 (8.9%) | 95 (5.8%) | 33 (2.0%) | 108 (6.7%) | 63 (3.9%) | 1,109 (68.3%) | 1,624 | ||
Double flap | |||||||||||
No | 33 | 38 | 143 | 94 | 33 | 108 | 62 | 1,059 | 1,570 (96.7%) | 16.105 | <0.001 |
Total | 33 (2.0%) | 39 (2.4%) | 144 (8.9%) | 95 (5.8%) | 33 (2.0%) | 108 (6.7%) | 63 (3.9%) | 1,109 (68.3%) | 1,624 | ||
Tracheostomy | |||||||||||
0 | 12 | 14 | 47 | 57 | 17 | 6 | 28 | 173 | 354 (21.8%) | 177.674 | <0.001 |
1 | 21 | 25 | 97 | 38 | 16 | 102 | 35 | 936 | 1,270 (78.2%) | ||
Total | 33 (2.0%) | 39 (2.4%) | 144 (8.9%) | 95 (5.8%) | 33 (2.0%) | 108 (6.7%) | 63 (3.9%) | 1,109 (68.3%) | 1,624 | ||
Radial forearm free flap | |||||||||||
No | 8 | 10 | 23 | 43 | 14 | 62 | 32 | 537 | 729 (44.9%) | 73.611 | <0.001 |
Yes | 25 | 29 | 121 | 52 | 19 | 46 | 31 | 572 | 895 (55.1%) | ||
Total | 33 (2.0%) | 39 (2.4%) | 144 (8.9%) | 95 (5.8%) | 33 (2.0%) | 108 (6.7%) | 63 (3.9%) | 1,109 (68.3%) | 1,624 | ||
Anterolateral thigh flap | |||||||||||
No | 33 | 38 | 143 | 67 | 26 | 78 | 49 | 950 | 1,384 (85.2%) | 67.83 | <0.001 |
Yes | 0 | 1 | 1 | 28 | 7 | 30 | 14 | 159 | 240 (14.8%) | ||
Total | 33 (2.0%) | 39 (2.4%) | 144 (8.9%) | 95 (5.8%) | 33 (2.0%) | 108 (6.7%) | 63 (3.9%) | 1,109 (68.3%) | 1,624 | ||
Fibula flap | |||||||||||
No | 31 | 30 | 138 | 84 | 29 | 99 | 50 | 997 | 1,458 (89.8%) | 21.601 | <0.001 |
Yes | 2 | 9 | 6 | 11 | 4 | 9 | 13 | 112 | 166 (10.2%) | ||
Total | 33 (2.0%) | 39 (2.4%) | 144 (8.9%) | 95 (5.8%) | 33 (2.0%) | 108 (6.7%) | 63 (3.9%) | 1,109 (68.3%) | 1,624 | ||
DCIA flap | |||||||||||
No | 31 | 39 | 132 | 94 | 32 | 91 | 63 | 1,041 | 1,523 (93.8%) | 29.695 | <0.001 |
Yes | 2 | 0 | 12 | 1 | 1 | 17 | 0 | 68 | 101 (6.2%) | ||
Total | 33 (2.0%) | 39 (2.4%) | 144 (8.9%) | 95 (5.8%) | 33 (2.0%) | 108 (6.7%) | 63 (3.9%) | 1,109 (68.3%) | 1,624 | ||
Scapula system | |||||||||||
No | 32 | 39 | 144 | 95 | 33 | 107 | 60 | 980 | 1,490 (91.7%) | 54.57 | <0.001 |
Yes | 1 | 0 | 0 | 0 | 0 | 1 | 3 | 129 | 134 (8.3%) | ||
Total | 33 (2.0%) | 39 (2.4%) | 144 (8.9%) | 95 (5.8%) | 33 (2.0%) | 108 (6.7%) | 63 (3.9%) | 1,109 (68.3%) | 1,624 | ||
No | 33 | 39 | 144 | 93 | 33 | 105 | 61 | 1,021 | 1,529 (94.2%) | 29.315 | <0.001 |
Yes | 0 | 0 | 0 | 2 | 0 | 3 | 2 | 88 | 95 (5.8%) | ||
Total | 33 (2.0%) | 39 (2.4%) | 144 (8.9%) | 95 (5.8%) | 33 (2.0%) | 108 (6.7%) | 63 (3.9%) | 1,109 (68.3%) | 1,624 | ||
Rectus abdominis | |||||||||||
No | 30 | 39 | 141 | 93 | 33 | 108 | 63 | 1,053 | 1,560 (96.1%) | 18.041 | 0.0118 |
Yes | 3 | 0 | 3 | 2 | 0 | 0 | 0 | 56 | 64 (3.9%) | ||
Total | 33 (2.0%) | 39 (2.4%) | 144 (8.9%) | 95 (5.8%) | 33 (2.0%) | 108 (6.7%) | 63 (3.9%) | 1,109 (68.3%) | 1,624 | ||
Other flap | |||||||||||
0 | 33 | 38 | 142 | 95 | 33 | 108 | 63 | 1,104 | 1,616 (99.5%) | 9.241 | 0.8153 |
1 | 0 | 1 | 2 | 0 | 0 | 0 | 0 | 5 | 7 (0.5%) | ||
Missing | 0 | 0 | 0 | 0 | 0 | 0 | 0 | 0 | 0 (0%) | ||
Total | 33 (2.0%) | 39 (2.4%) | 144 (8.9%) | 95 (5.8%) | 33 (2.0%) | 108 (6.7%) | 63 (3.9%) | 1,109 (68.3%) | 1,624 | ||
Flap failure | |||||||||||
No | 31 | 35 | 133 | 89 | 31 | 102 | 58 | 1,038 | 1,517 (93.4%) | 5.682 | 0.9739 |
Yes | 2 | 3 | 6 | 8 | 1 | 3 | 3 | 50 | 76 (4.7%) | ||
Partial | 0 | 1 | 5 | 1 | 1 | 3 | 2 | 21 | 34 (2.1%) | ||
Total | 33 (2.0%) | 39 (2.4%) | 144 (8.9%) | 98 (6%) | 33 (2.0%) | 108 (6.7%) | 63 (3.9%) | 1,109 (68.3%) | 1,624 |
These variables were studied in WEKA platform undertaking exploratory analyses using the following algorithms; logistic regression, naïve Bayes, J48 decision tree, random forests and an artificial neural network. The outcome was binary, namely failure versus no failure, by excluding cases with partial failure. The models showed weak discrimination (C statistic <0.7) suggesting free flap failure, which is a relatively rare event (<5%) will need more data to model effectively. The best model was a simple BayesNetwork, ROC (C-statistic 0.66) on 10-fold cross validation. The specificity was low (0.11) and this was improved with reducing the cut-off from 0.5 to 0.1 with a reduction in sensitivity (0.83) but an improved specificity (0.47) and overall accuracy of (0.81). The model predicted nearly 50% of free flap failures. The predicted probabilities were tested within the logistic regression analyses in MedCalc, and the ROC C-statistic for the entire cohort was (0.71) which is over-optimistic (Table S4). The calibration plot is shown (Figure 1) demonstrating acceptable performance (Hosmer-Lemeshow Goodness of fit λ2 6.9, P=0.53).
The entire dataset was divided into the respective hospitals and raw flap failure data was used to develop CuSUM against time (Figure 2A,B,C,D,E,F). The predicted probabilities were used to give patient-specific risks to modify the CuSUM chart. The risk-adjusted CUSUM chart plots the function:
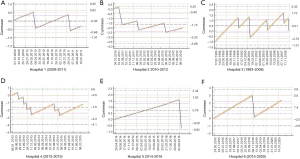
where Wt is a weight assigned to each value of t. In our study, the risk-adjusted CuSUM charts were updated for every patient thus each value of t corresponds to a subsequent patient care episode. Consequently, the weights Wt are given by
Here, Yt is the outcome of a patient care episode, t (free flap failure within 30 days of operation date yes/no) and pt is the expected probability of the free flap failure estimated from a prediction model based on the audit data from each hospital. Finally, RA >1 is a specified odds ratio (OR) increase in the outcome rate, as compared to the reference period, that the risk-adjusted CuSUM chart is set to detect, and we set it at 2 (or twice the expected rate). We set the weight Wt as positive if the patient did not have the outcome, and negative if they did. The absolute value of the weight was large if the outcome is unexpected. Thus, in our study, if more patients had free tissue failure than predicted, the CuSUM function would decrease. The risk-adjusted CuSUM for the largest cohort (Hospital 8) is shown (Figure 3).
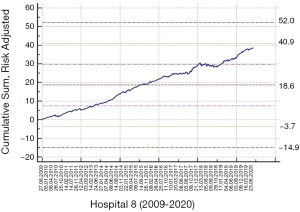
Discussion
Metric selection is key to effective monitoring of surgical units performance. Whilst face-validity is a component of good metric selection, it is subjective and the implication is the metrics hold face-validity for the surgical members of the team. We argue a critical aspect of metric choice is underplayed, namely the ability to risk-adjust a metric to account for complexity of care.
We are aware of risk adjusted CuSUM charts in routine use now in the National Emergency Laparotomy Audit (13)where clinical teams can enter information on an online dashboard seeing recent mortality in the context of live unit level data and national (aggregated) data. We judge such live feedback, whilst carrying a novelty value initially, essentially serves to strengthen the link between treating teams and their surgical speciality in a way that (hopefully) improves engagement sustainably. We suspect charting free flap success vs. failure in this way may be achievable. Highlighting the risk adjusted CuSUM chart (Figure 2F) suggests unusual deterioration in performance in November 2018 which, though not breaching the 3sd alarm limit, comes close to meriting departmental scrutiny of surgeon, patient and ward factors. As this model is in its development, we have not explored alternative alarm limits beyond 2nd or 3rd standard deviation (SD), such as bootstrapping methods discussed by Rasmussen (13). Automatic resets to baseline can be implemented after 3rd SD alarm level breach, and we suggest that this could be done every 6 months, or every 50 flap successes, which ever occurs sooner. This is a clinical decision and seeks to avoid the pitfall of cumulative good performance obscuring a significant deterioration, as seen (Figure 2F and Figure 3).
Free tissue transfer failure rates varied between hospitals, though not to a significant degree (3–8%, mean 4.7%). The implication of this non-significant difference is however profound in terms of risk to the patient of further complications, patient experience and hospital resource allocation. Regarding hospital resource allocation, in the UK health system these charges are born by the taxpayer and financial matters do not frame the clinical decisions relating to patient care at the patient level. The same is not seen in the US and many other modern health care systems where academic studies explicitly relate cost of care to post-operative events secondary to free tissue transfer (14). In the UK, if cost of care is to be understood, it is at the level of health commissioners seeking data on which to base judgements about where to focus purchase of care at a regional level, based on evidence of good outcomes and engagement in quality improvement initiatives and national audit.
We are aware of a more detailed classification of free-tissue transfer that can report more effectively on issues of resource allocation and patient-pertinent factors (15) but a decision was made at an early stage, as partial flap failure was a rare event (2%), modelling the sub-category outcomes of this group was untenable.
This paper has summarised the performance of different algorithms for predicting outcomes, using pre-operative data alone, including 30-day complications, 30-day severe complications, length of hospital stay >14 days and positivity of surgical margins. We presented a new risk-adjustment algorithm for predicting free tissue transfer failure and embedded that into a CuSUM control chart to demonstrate its potential utility as a live-audit tool for the purpose of contemporaneous assessment of surgical performance within a Head & Neck unit offering microvascular reconstructive treatments. Together these form the basis of a growing system of metrics that provide a ‘clinical-care signature’ that informs the treating teams to allow learning and development within a robust clinical governance framework. It also, if presented transparently, assures commissioners and public about quality of care.
Acknowledgments
Funding: None.
Footnote
Provenance and Peer Review: This article was commissioned by the editorial office, Frontiers of Oral and Maxillofacial Medicine, for the series “Head and Neck Reconstruction”. The article has undergone external peer review.
Conflicts of Interest: The authors have completed the ICMJE uniform disclosure form (available at https://fomm.amegroups.com/article/view/10.21037/fomm-20-89/coif). The series “Head and Neck Reconstruction” was commissioned by the editorial office without any funding or sponsorship. MH served as the unpaid Guest Editor of the series, and serves as an unpaid editorial board member of Frontiers of Oral and Maxillofacial Medicine from October 2019 to September 2021. DFT reports grants from East Kent Hospitals Research and Innovation Grant, during the conduct of the study. The authors have no other conflicts of interest to declare.
Ethical Statement: The authors are accountable for all aspects of the work in ensuring that questions related to the accuracy or integrity of any part of the work are appropriately investigated and resolved.
Open Access Statement: This is an Open Access article distributed in accordance with the Creative Commons Attribution-NonCommercial-NoDerivs 4.0 International License (CC BY-NC-ND 4.0), which permits the non-commercial replication and distribution of the article with the strict proviso that no changes or edits are made and the original work is properly cited (including links to both the formal publication through the relevant DOI and the license). See: https://creativecommons.org/licenses/by-nc-nd/4.0/.
References
- Learning from Bristol. The Report of the Public Inquiry into children’s heart surgery at the Bristol Royal Infirmary 1984-1995. Presented to Parliament by Ian Kennedy QC. Available online: https://webarchive.nationalarchives.gov.uk/20090811143822
- Birkmeyer JD, Dimick JB, Birkmeyer NJ. Measuring the quality of surgical care: structure, process, or outcomes? J Am Coll Surg 2004;198:626-32. [Crossref] [PubMed]
- Roques F, Michel P, Goldstone AR, et al. The logistic EuroSCORE. Eur Heart J 2003;24:881-2. [Crossref] [PubMed]
- Medical Algorithms List. UK. 2020. Available online: https://www.medicalalgorithms.com/
- Graboyes EM, Gross J, Kallogjeri D, et al. Association of Compliance With Process-Related Quality Metrics and Improved Survival in Oral Cavity Squamous Cell Carcinoma. JAMA Otolaryngol Head Neck Surg 2016;142:430-7. [Crossref] [PubMed]
- Comparative Study on Classic Machine learning Algorithms. Medium: Towards data science. US. Available online: https://towardsdatascience.com/comparative-study-on-classic-machine-learning-algorithms-24f9ff6ab222
- Bayes T, Price R. An Essay towards Solving a Problem in the Doctrine of Chances. By the Late Rev. Mr. Bayes, F. R. S. Communicated by Mr. Price, in a Letter to John Canton, A. M. F. R. S. Philosophical Transactions of the Royal Society of London 1763;53:370-418. [Crossref]
- Dindo D, Demartines N, Clavien PA. Classification of surgical complications: a new proposal with evaluation in a cohort of 6336 patients and results of a survey. Ann Surg 2004;240:205-13. [Crossref] [PubMed]
- The Royal College of Pathologists. Dataset for histopathology reporting of nodal excisions and neck dissection specimens associated with head and neck carcinomas. London: The Royal College of Pathologists, 2013 and 2014. Available online: https://www.rcpath.org/resource-libraryhomepage/publications/cancer-datasets.html
- Tighe D, Lewis-Morris T, Freitas A. Machine learning methods applied to audit of surgical outcomes after treatment for cancer of the head and neck. Br J Oral Maxillofac Surg 2019;57:771-7. [Crossref] [PubMed]
- Tighe D, Fabris F, Freitas A. Machine learning methods applied to audit of surgical margins after curative surgery for head and neck cancer. Br J Oral Maxillofac Surg 2021;59:209-16. [Crossref] [PubMed]
- Tighe DF, Thomas AJ, Sassoon I, et al. Developing a risk stratification tool for audit of outcome after surgery for head and neck squamous cell carcinoma. Head Neck 2017;39:1357-63. [Crossref] [PubMed]
- Rasmussen TB, Ulrichsen SP, Nørgaard M. Use of risk-adjusted CUSUM charts to monitor 30-day mortality in Danish hospitals. Clin Epidemiol 2018;10:445-56. [Crossref] [PubMed]
- Sweeny L, Rosenthal EL, Light T, et al. Outcomes and cost implications of microvascular reconstructions of the head and neck. Head Neck 2019;41:930-9. [Crossref] [PubMed]
- Ho MW, Nugent M, Puglia F, et al. Results of flap reconstruction: categorisation to reflect outcomes and process in the management of head and neck defects. Br J Oral Maxillofac Surg 2019;57:935-7. [Crossref] [PubMed]
Cite this article as: Tighe DF, McMahon J, Ho M, Sassoon I. Risk adjustment in audit of outcome after head and neck surgery applied to cumulative sum chart methodology to monitor of free flap failure. Front Oral Maxillofac Med 2022;4:5.